Invited Talks
Machine learning in health has made impressive progress in recent years, powered by an increasing availability of health-related data and high-capacity models. While many models in health now perform at, or above, humans in a range of tasks across the human lifespan, models also learn societal biases and may replicate or expand them. In this talk, Dr. Marzyeh Ghassemi will focus on the need for machine learning researchers and model developers to create robust models that can be ethically deployed in health settings, and beyond. Dr. Ghassemi's talk will span issues in data collection, outcome definition, algorithm development, and deployment considerations.
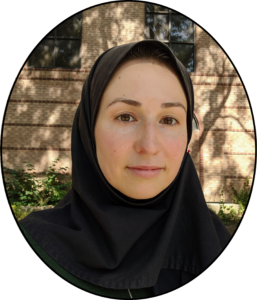
Marzyeh Ghassemi
Dr. Marzyeh Ghassemi is an Assistant Professor at MIT in Electrical Engineering and Computer Science (EECS) and Institute for Medical Engineering & Science (IMES), and a Vector Institute faculty member holding a Canadian CIFAR AI Chair and Canada Research Chair. She holds MIT affiliations with the Jameel Clinic and CSAIL.
Professor Ghassemi holds a Herman L. F. von Helmholtz Career Development Professorship, and was named a CIFAR Azrieli Global Scholar and one of MIT Tech Review’s 35 Innovators Under 35. Previously, she was a Visiting Researcher with Alphabet’s Verily. She is currently on leave from the University of Toronto Departments of Computer Science and Medicine. Prior to her PhD in Computer Science at MIT, she received an MSc. degree in biomedical engineering from Oxford University as a Marshall Scholar, and B.S. degrees in computer science and electrical engineering as a Goldwater Scholar at New Mexico State University.
This talk talk has a single objective: to advocate for machine learning infused with social purpose. Social purpose here is an invitation to deepen our inquiries as investigators and inventors into the relationships between machine learning, our planet, and each other. In this way, social purpose transforms our field of machine learning: into something that is both technical and social. And my belief is that machine learning with social purpose will provide the passion and momentum for the contributions that are needed in overcoming the myriad of global challenges and in achieving our global goals. To make this all concrete, the talk will have three parts: machine learning for the Earth systems, sociotechnical AI, and strengthening global communities. And we’ll cover topics on generative models; evaluations and experts; healthcare and climate; fairness, ethics and safety; and bias and global inclusion. By the end, I hope we’ll have set the scene for a rich discussion on our responsibility and agency as researchers, and new ways of driving machine learning with social purpose.
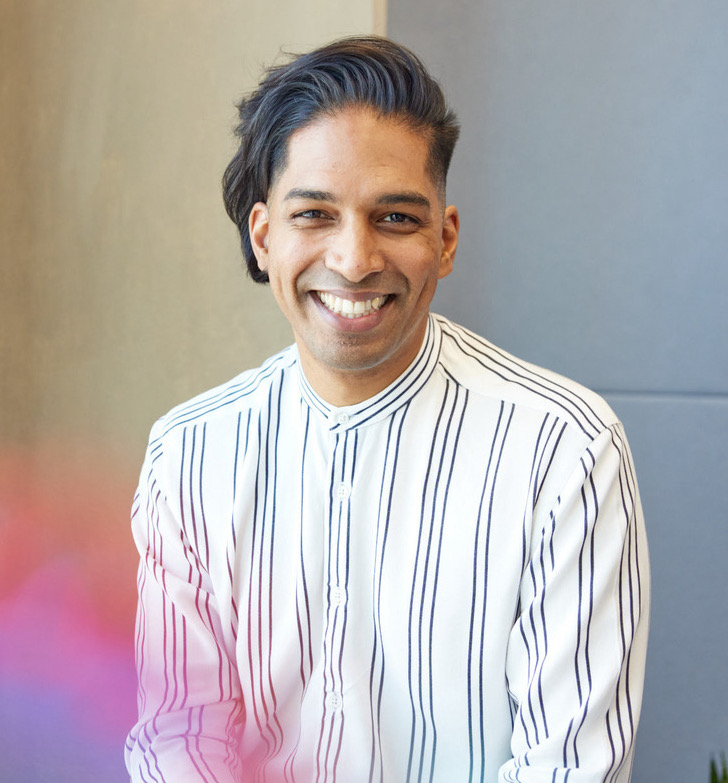
Shakir Mohamed
Shakir Mohamed works on technical and sociotechnical questions in machine learning research, working on problems in machine learning principles, applied problems in healthcare and environment, and ethics and diversity. Shakir is a Director for Research at DeepMind in London, an Associate Fellow at the Leverhulme Centre for the Future of Intelligence, and an Honorary Professor of University College London. Shakir is also a founder and trustee of the Deep Learning Indaba, a grassroots charity whose work is to build pan-African capacity and leadership in AI. Amongst other roles, Shakir served as the senior programme chair for ICLR 2021, and as the General Chair for NeurIPS 2022. Shakir also serves on the Board of Directors for some of the leading conferences in the field of machine learning and AI (ICML, ICLR, NeurIPS), is a member of the Royal Society diversity and inclusion committee, and on the international scientific advisory committee for the pan-Canadian AI strategy. Shakir is from South Africa, completed a postdoc at the University of British Columbia, received his PhD from the University of Cambridge, and received his masters and undergraduate degrees in Electrical and Information engineering from the University of the Witwatersrand, Johannesburg.
Jennifer A. Doudna, Innovative Genomics Institute, Howard Hughes Medical Institute and University of California Berkeley & UCSF/Gladstone Institutes Machine learning will have profound impacts on biological research in ways that are just beginning to be imagined. The intersection of ML and CRISPR provides exciting examples of the opportunities and challenges in fields ranging from healthcare to climate change. CRISPR-Cas programmable proteins can edit specific DNA sequences in cells and organisms, generating new biological insights as well as approved therapeutics and improved crops. I will discuss how ML may accelerate and perhaps fundamentally alter our use of CRISPR genome editing in both humans and microbes.
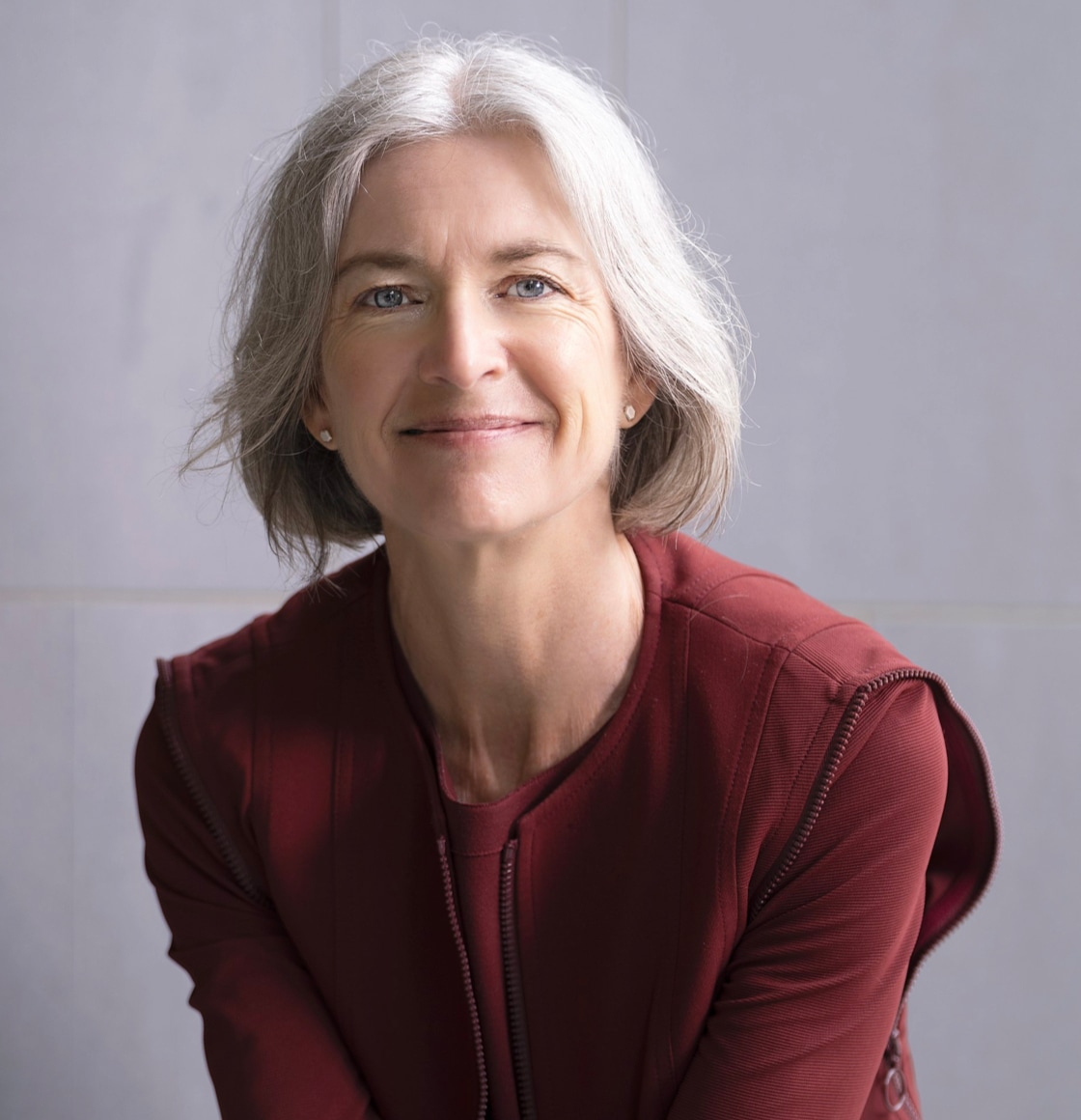
Jennifer Doudna
Jennifer Doudna, PhD is a biochemist at the University of California, Berkeley. Her groundbreaking development of CRISPR-Cas9 — a genome engineering technology that allows researchers to edit DNA — with collaborator Emmanuelle Charpentier earned the two the 2020 Nobel Prize in Chemistry and forever changed the course of human and agricultural genomics research. She is also the Founder of the Innovative Genomics Institute, the Li Ka Shing chancellor’s chair in Biomedical and Health Sciences, and a member of the Howard Hughes Medical Institute, Lawrence Berkeley National Lab, Gladstone Institutes, the National Academy of Sciences, and the American Academy of Arts and Sciences. She is a leader in the global public debate on the responsible use of CRISPR and has co-founded and serves on the advisory panel of several companies that use the technology in unique ways. Doudna is the co-author of “A Crack in Creation,” a personal account of her research and the societal and ethical implications of gene editing. Learn more at innovativegenomics.org/jennifer-doudna.
Proxy objectives are a fundamental concept in machine learning. That is, there's a true objective that we care about, but it's hard to compute or estimate, so instead we construct a locally-valid approximation and optimize that. I will examine reinforcement from human feedback with this lens, as a chain of approximations, each of which can widen the gap between the desired and achieved result.
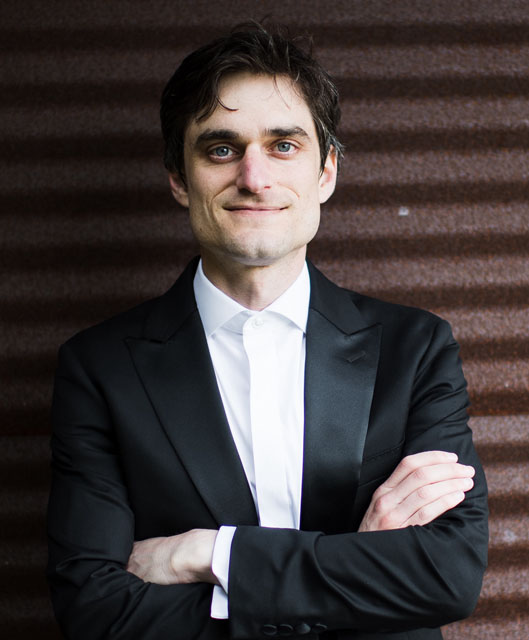
John Schulman
John now leads a team working on ChatGPT and RL from Human Feedback at OpenAI, where he was a cofounder. His recent published work includes combining language models with retrieval (WebGPT) and scaling laws of RL and alignment. Earlier he developed some of the foundational methods of deep RL (TRPO, PPO). Before OpenAI, John got a PhD from UC Berkeley, advised by Pieter Abbeel. In his free time, he enjoys running, jazz piano, and raising chickens.