Organizers
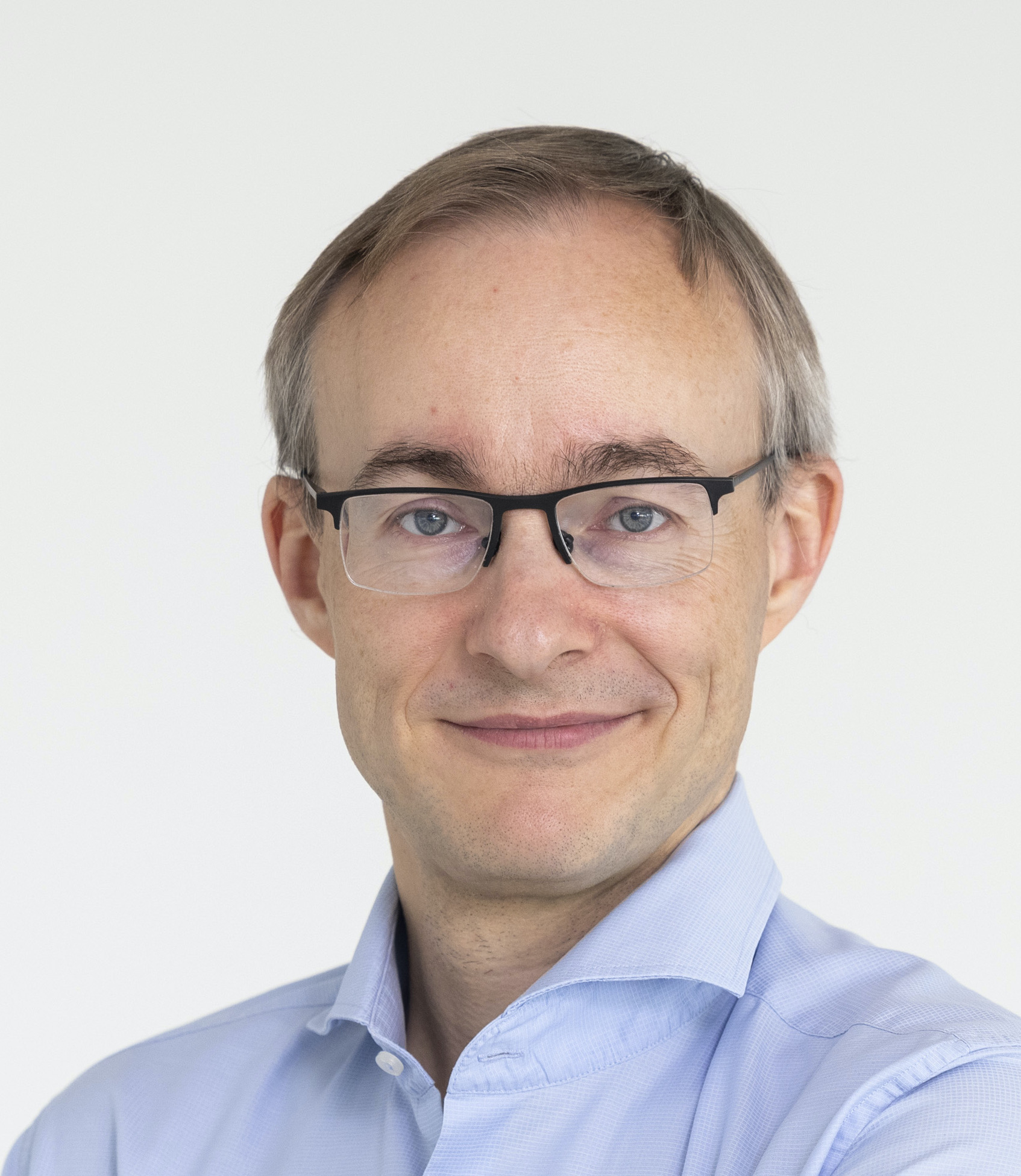
Bio
Andreas Krause is a Professor of Computer Science at ETH Zurich, where he leads the Learning & Adaptive Systems Group. He also serves as Academic Co-Director of the Swiss Data Science Center and Chair of the ETH AI Center, and co-founded the ETH spin-off LatticeFlow. Before that he was an Assistant Professor of Computer Science at Caltech. He received his Ph.D. in Computer Science from Carnegie Mellon University (2008) and his Diplom in Computer Science and Mathematics from the Technical University of Munich, Germany (2004). He is a Max Planck Fellow at the Max Planck Institute for Intelligent Systems, an ELLIS Fellow, a Microsoft Research Faculty Fellow and a Kavli Frontiers Fellow of the US National Academy of Sciences. He received the Rössler Prize, ERC Starting Investigator and ERC Consolidator grants, the German Pattern Recognition Award, an NSF CAREER award as well as the ETH Golden Owl teaching award. His research has received awards at several premier conferences and journals, including the ACM SIGKDD Test of Time award 2019 and the ICML Test of Time award 2020. Andreas Krause served as Program Co-Chair for ICML 2018, and currently serves as General Chair for ICML 2023 and as Action Editor for …

Bio
Barbara E. Engelhardt is a professor at Stanford University. She joined from Princeton, where she was a professor from 2014-2022, and previously Duke University, where she was an assistant professor from 2011-2014. She graduated from Stanford University and received her Ph.D. from the University of California, Berkeley, advised by Professor Michael Jordan. She did postdoctoral research at the University of Chicago, working with Professor Matthew Stephens, and three years at Duke University as an assistant professor. Interspersed among her academic experiences, she spent two years working at the Jet Propulsion Laboratory, a summer at Google Research, and a year at 23andMe, a DNA ancestry service. Professor Engelhardt received an NSF Graduate Research Fellowship, the Google Anita Borg Memorial Scholarship, and the Walter M. Fitch Prize from the Society for Molecular Biology and Evolution. As a faculty member, she received the NIH NHGRI K99/R00 Pathway to Independence Award, a Sloan Faculty Fellowship, and an NSF CAREER Award. Professor Engelhardt’s research interests involve developing statistical models and methods for the analysis of high-dimensional biomedical data, with a goal of understanding the underlying biological mechanisms of complex phenotypes and human disease.
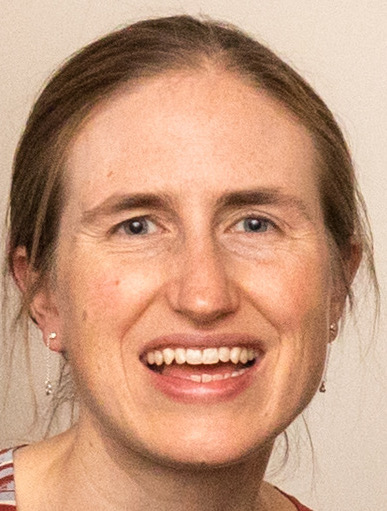
Bio
Emma Brunskill is an associate tenured professor in the Computer Science Department at Stanford University. Brunskill’s lab aims to create AI systems that learn from few samples to robustly make good decisions and is part of the Stanford AI Lab, the Stanford Statistical ML group, and AI Safety @Stanford. Brunskill has received a NSF CAREER award, Office of Naval Research Young Investigator Award, a Microsoft Faculty Fellow award and an alumni impact award from the computer science and engineering department at the University of Washington. Brunskill and her lab have received multiple best paper nominations and awards both for their AI and machine learning work (UAI best paper, Reinforcement Learning and Decision Making Symposium best paper twice) and for their work in Ai of education (Intelligent Tutoring Systems Conference, Educational Data Mining conference x3, CHI).
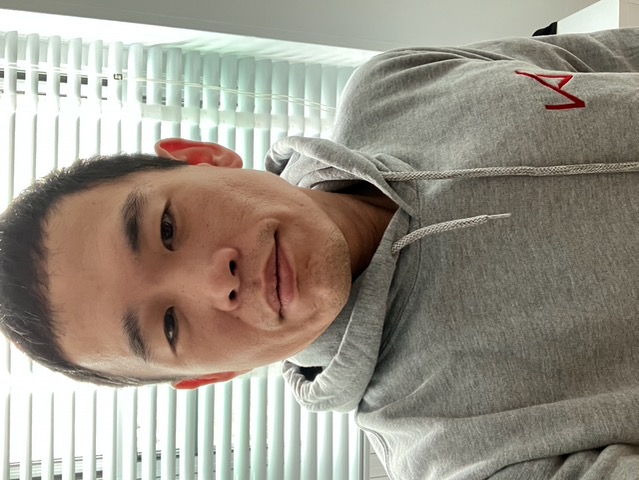
Bio
Kyunghyun Cho is an associate professor of computer science and data science at New York University and CIFAR Fellow of Learning in Machines & Brains. He is also a senior director of frontier research at the Prescient Design team within Genentech Research & Early Development (gRED). He was a research scientist at Facebook AI Research from June 2017 to May 2020 and a postdoctoral fellow at University of Montreal until Summer 2015 under the supervision of Prof. Yoshua Bengio, after receiving MSc and PhD degrees from Aalto University April 2011 and April 2014, respectively, under the supervision of Prof. Juha Karhunen, Dr. Tapani Raiko and Dr. Alexander Ilin. He received the Samsung Ho-Am Prize in Engineering in 2021. He tries his best to find a balance among machine learning, natural language processing, and life, but almost always fails to do so.
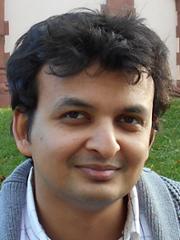
Bio
Adish Singla is a faculty member at the Max Planck Institute for Software Systems (MPI-SWS), Germany, where he has been leading the Machine Teaching Group since 2017. He conducts research in the area of Machine Teaching, with a particular focus on open-ended learning and problem-solving domains. In recent years, his research has centered around developing AI-driven educational technology for introductory programming environments. He has received several awards for his research, including an AAAI Outstanding Paper Honorable Mention Award (2022) and an ERC Starting Grant (2021). He also has extensive experience working in the industry and is a recipient of several industry awards, including a research grant from Microsoft Research Ph.D. Scholarship Programme (2018), Facebook Graduate Fellowship (2015), Microsoft Tech Transfer Award (2011), and Microsoft Gold Star Award (2010).
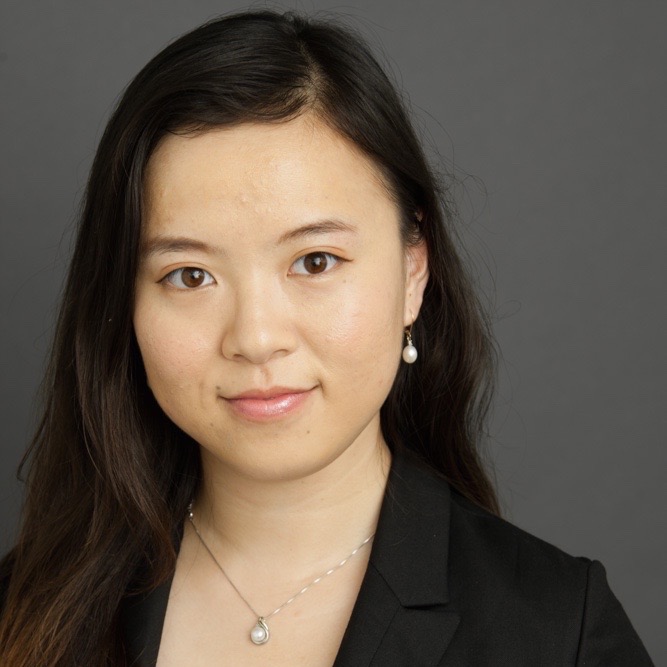
Bio
Dr. Rose Yu is an assistant professor at the University of California San Diego, Department of Computer Science and Engineering. She earned her Ph.D. in Computer Sciences at USC in 2017. She was subsequently a Postdoctoral Fellow at Caltech. Her research focuses on advancing machine learning techniques for large-scale spatiotemporal data analysis, with applications to sustainability, health, and physical sciences. A particular emphasis of her research is on physics-guided AI which aims to integrate first principles with data-driven models. Among her awards, she has won NSF CAREER Award, Faculty Research Award from JP Morgan, Facebook, Google, Amazon, and Adobe, Several Best Paper Awards, Best Dissertation Award at USC, and was nominated as one of the ’MIT Rising Stars in EECS’.
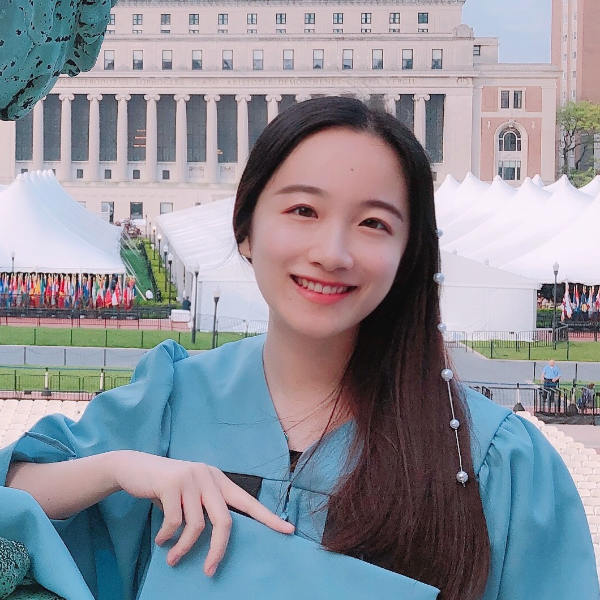
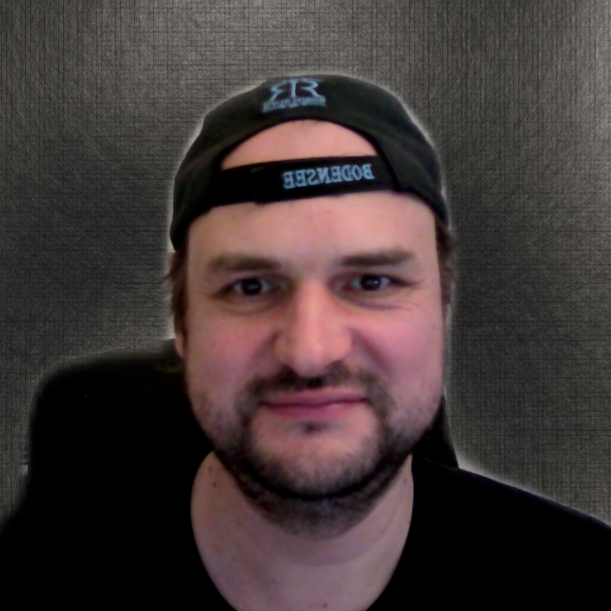
Bio
Currently, I am working as a Researcher in Information Visualisation, Visual Analytics, and Machine Learning at IBM Research AI in Cambridge, MA. I am interested in Visualization of large data sets of unstructured/semi-structured data, biological data, and neural network models. I enjoy advising students and enable them to do great work.
I try to be a good citizen in the community by reviewing regularly (InfoVis 2010-2018, BioVis 2012/13, CHI 2011/2014/2017-18, VAST 2010-2018, EuroVis,...), participating in InfoVis PC 2017-19, the VIS OC 2017-19, the BioVis 2013 OC and PC, and other committees. I had the honor to attend three great and motivating Dagstuhl seminars on InfoVis, BioVis, and Progressive Data Science. Oh, and I like to give talks from time to time.
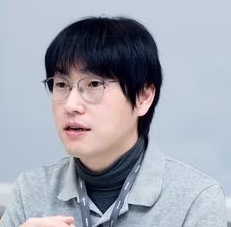
Bio
Jung-Woo Ha got his BS and PhD degrees in computer science from Seoul National University in 2004 and 2015. He got the 2014 Fall semester outstanding PhD dissertation award from Computer Science Dept. of Seoul National University. He worked as a research scientist and tech lead at NAVER LABS and research head of NAVER CLOVA. Currently, he works as the head of NAVER AI Lab in NAVER Cloud. He has contributed to the AI research community as Datasets and Benchmarks Co-chair for NeurIPS and Social Co-chair for ICML 2023 and NeurIPS 2022. Also, he has joined a senior technical program committee member, such as, Area chair for NeurIPS 2023 and 2022, Area chair for ICML 2023, and Senior area chair for COLING. His research interests include large language models, generative models, multimodal representation learning and their practical applications for real-world problems. In particular, he has mainly focused on practical task definition and evaluation protocol for continual learning in various domains.
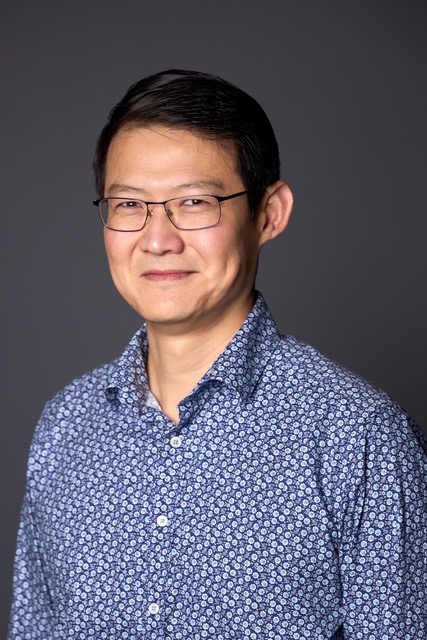
Bio
I am a senior principal research scientist at the Machine Learning Research Group, Data61, CSIRO, and the director of the machine learning and artificial intelligence future science platform at CSIRO. I am also an adjunct associate professor at the Australian National University. I am interested in enabling scientific discovery by extending statistical machine learning methods. In recent years, we have developed new optimisation methods for solving problems such as ranking, feature selection and experimental design, with the aim of solving scientific questions in collaboration with experts in other fields. This has included diverse problems in genomics, systems biology, and astronomy. I advocate strongly for open science, as well as diversity and inclusion.
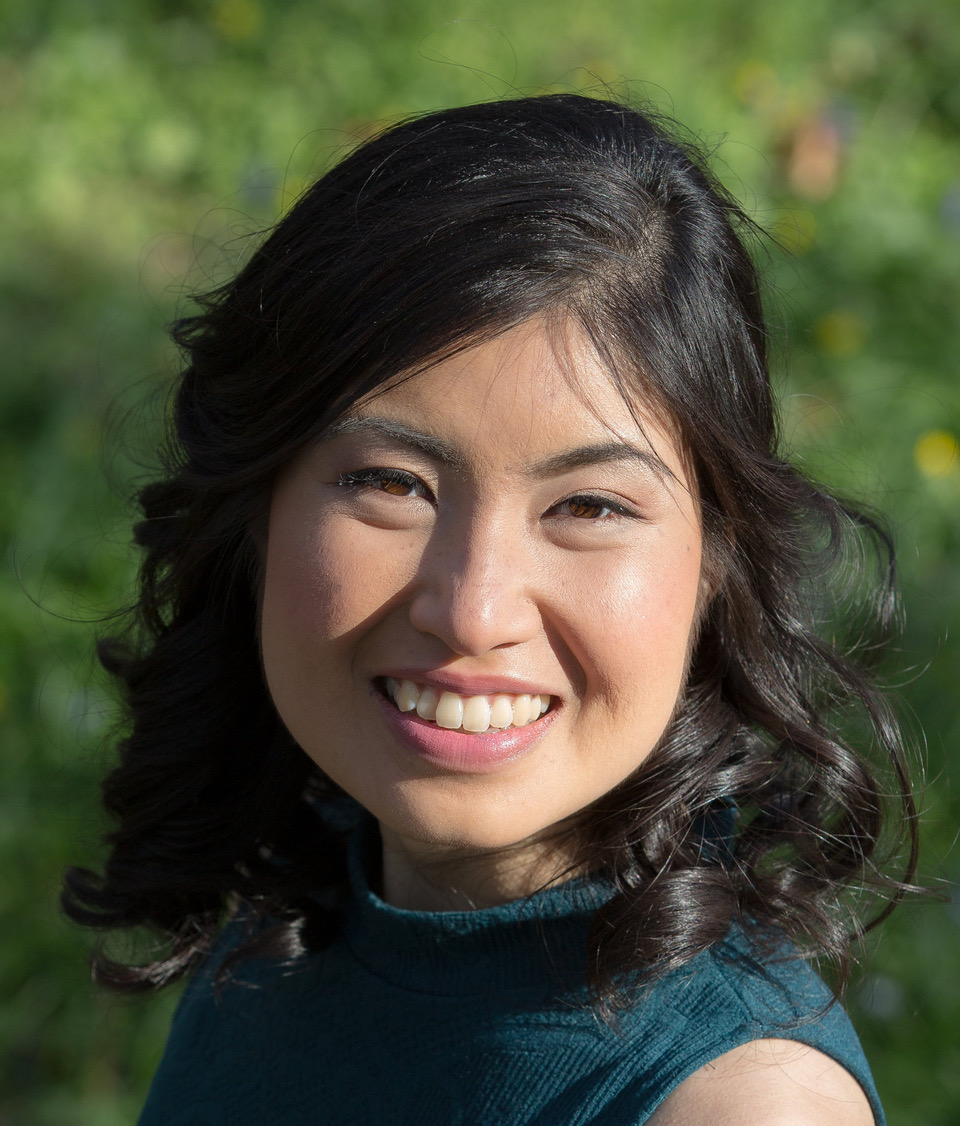
Bio
Po-Ling Loh received her PhD in Statistics from UC Berkeley in 2014. From 2014-2016, she was an Assistant Professor of Statistics at the University of Pennsylvania. From 2016-2018, she was an Assistant Professor of Electrical & Computer Engineering at the University of Wisconsin-Madison, and from 2019-2020, she was an Associate Professor of Statistics at the University of Wisconsin-Madison and a Visiting Associate Professor of Statistics at Columbia University. She joined the Department of Pure Mathematics and Mathematical Statistics at the University of Cambridge in 2021, where she is currently a Professor of Statistics. Loh’s current research interests include high-dimensional statistics, robustness, and differential privacy. She is a recipient of an NSF CAREER Award, an Army Research Office Young Investigator Award, an Institute of Mathematical Statistics Tweedie New Researcher Award, a Bernoulli Society New Researcher Award, and a Hertz Fellowship.
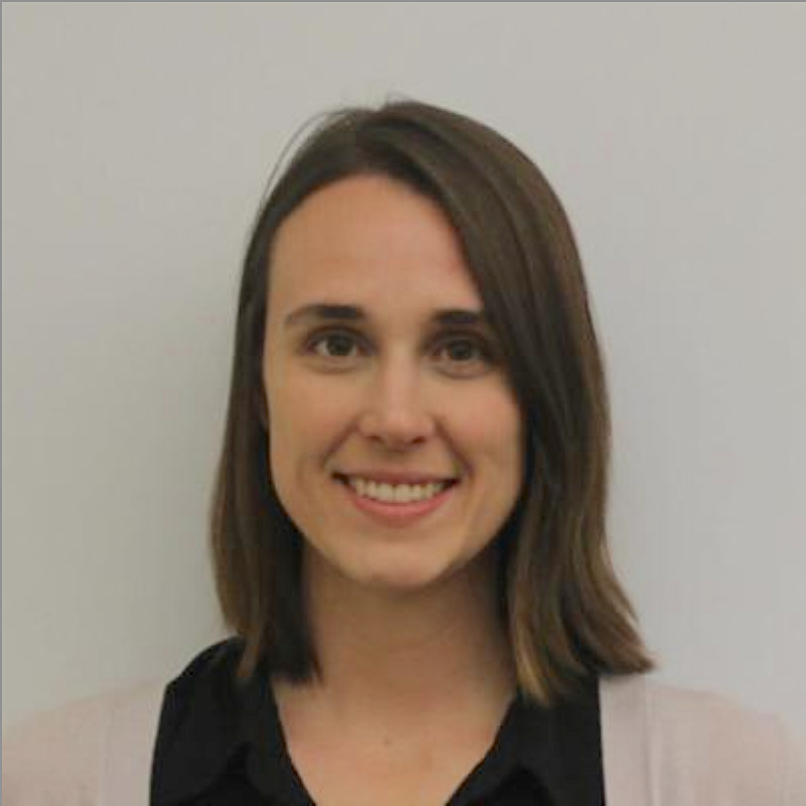
Bio
Virginia Smith is an assistant professor in the Machine Learning Department at Carnegie Mellon University, and a courtesy faculty member in the Electrical and Computer Engineering Department. Her research interests span machine learning, optimization, and distributed systems. Prior to CMU, Virginia was a postdoc at Stanford University, received a Ph.D. in Computer Science from UC Berkeley, and obtained undergraduate degrees in Mathematics and Computer Science from the University of Virginia.
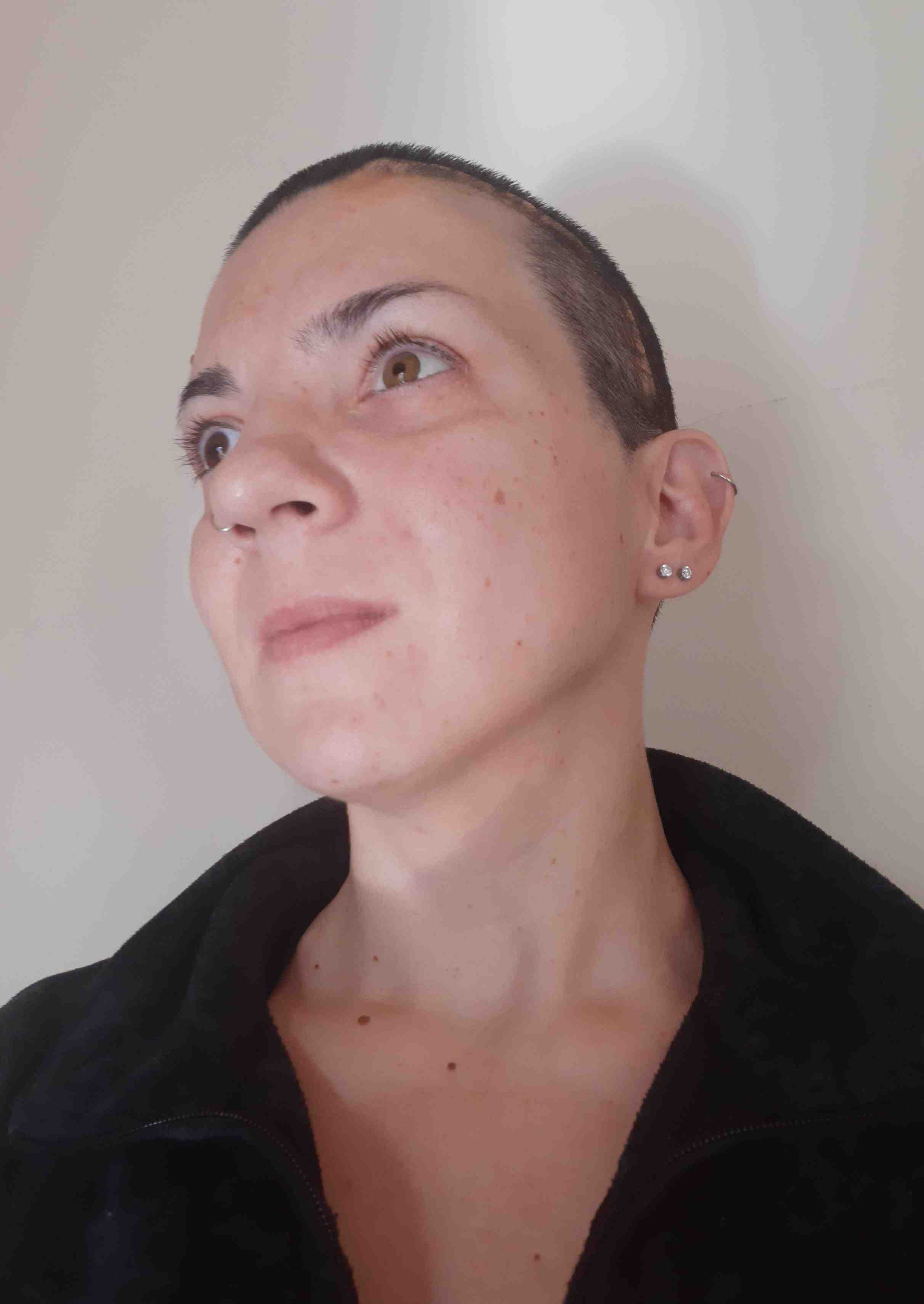
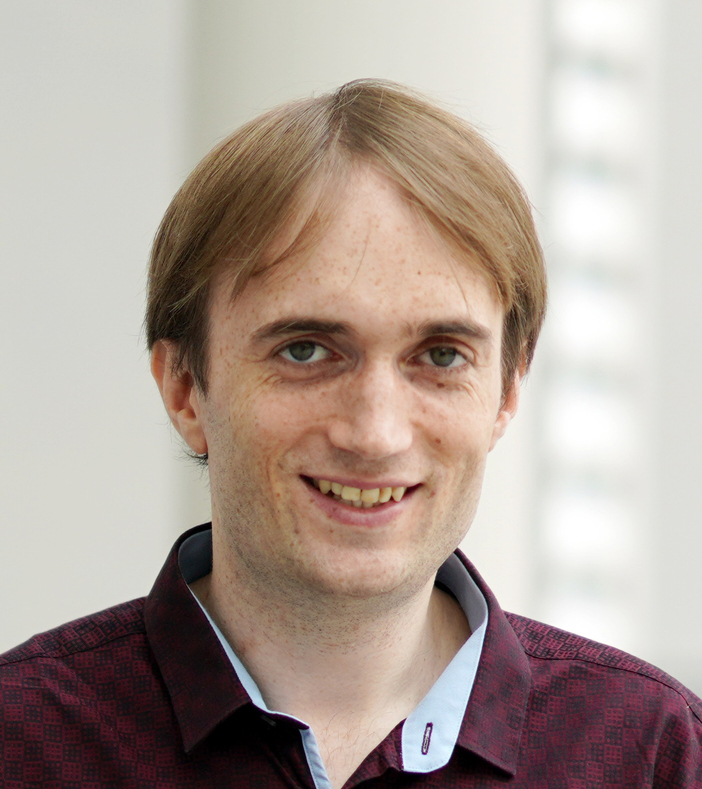
Bio
Jonathan is an assistant professor in the Department of Computer Science and Department of Mathematics at NUS. His research interests are in information theory, machine learning, and high-dimensional statistics, particularly their intersection. He is a holder of the Singapore National Research Foundation (NRF) fellowship and the NUS Presidential Young Professorship. Previously, he did his post-doc at EPFL and his PhD at the University of Cambridge.
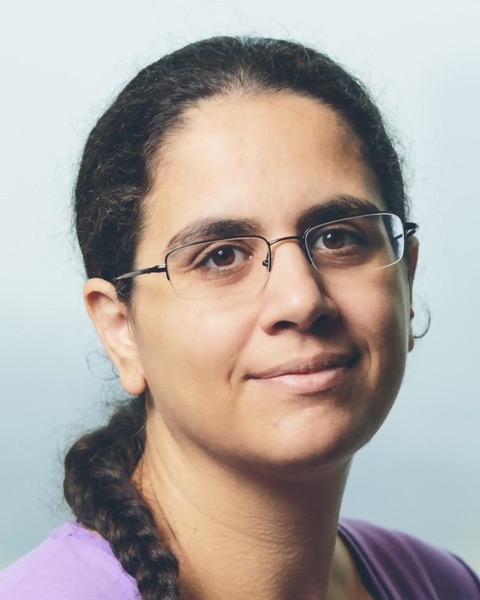
Bio
Sivan Sabato is an Associate Professor at the Department of Computer Science at Ben Gurion University of the Negev. Before that, she was a post-doctoral fellow at Microsoft Research New England. She received her Ph.D. from the School of Computer Science and Engineering at the Hebrew University of Jerusalem. Prof. Sabato is a recipient of the Alon Scholarship of the Council for Higher Education of Israel for excellent new faculty. She is also a recipient of the Google Anita Borg Memorial Scholarship, and an alumna of the Adams Fellowship Program of the Israel Academy of Sciences and Humanities.
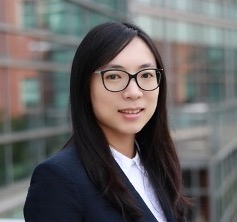
Bio
Dr. Bo Li is an assistant professor in the Department of Computer Science at the University of Illinois at Urbana–Champaign. She is the recipient of the IJCAI Computers and Thought Award, Alfred P. Sloan Research Fellowship, AI’s 10 to Watch, NSF CAREER Award, MIT Technology Review TR-35 Award, Dean's Award for Excellence in Research, C.W. Gear Outstanding Junior Faculty Award, Intel Rising Star award, Symantec Research Labs Fellowship, Rising Star Award, Research Awards from Tech companies such as Amazon, Facebook, Intel, IBM, and eBay, and best paper awards at several top machine learning and security conferences. Her research focuses on both theoretical and practical aspects of trustworthy machine learning, which is at the intersection of machine learning, security, privacy, and game theory. She has designed several scalable frameworks for trustworthy machine learning and privacy-preserving data publishing. Her work has been featured by major publications and media outlets such as Nature, Wired, Fortune, and New York Times.
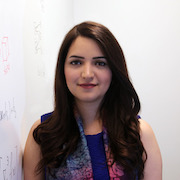
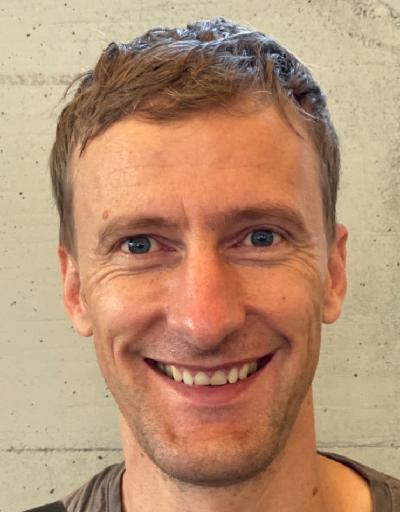
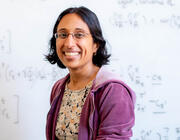
Bio
Finale Doshi-Velez is a Gordon McKay Professor in Computer Science at the Harvard Paulson School of Engineering and Applied Sciences. She completed her MSc from the University of Cambridge as a Marshall Scholar, her PhD from MIT, and her postdoc at Harvard Medical School. Her interests lie at the intersection of machine learning, healthcare, and interpretability.
Selected Additional Shinies: BECA recipient, AFOSR YIP and NSF CAREER recipient; Sloan Fellow; IEEE AI Top 10 to Watch
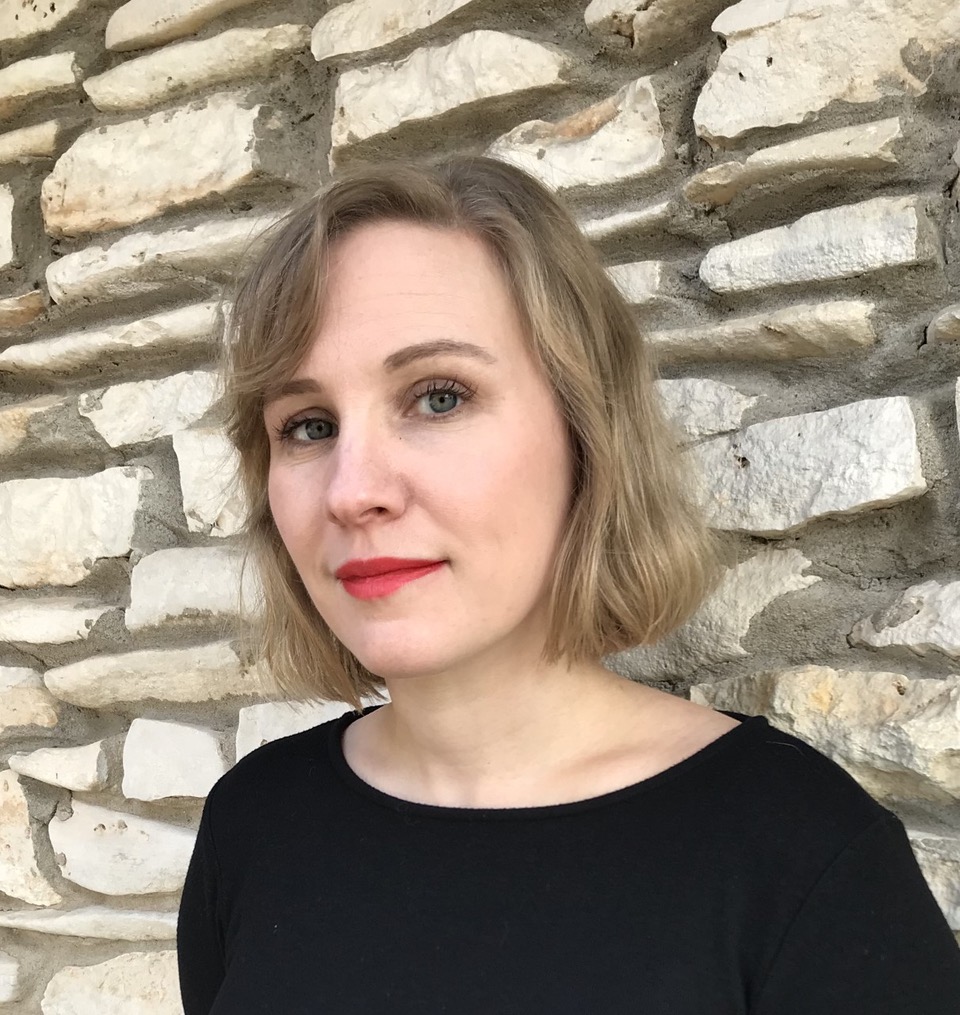
Bio
Sinead Williamson is a Machine Learning Researcher at Apple and an Associate Professor of Statistics at the University of Texas at Austin. She works primarily on Bayesian methods for machine learning, with particular interests in Bayesian nonparametrics, scalable sampling methods, and modeling structured data with complex dependency structures. She has served as Diversity and Inclusion chair for AISTATS, Newcomer’s Chair for ICML, chair of the ISBA program committee, and as a member of the Board of Directors of Women in Machine Learning.
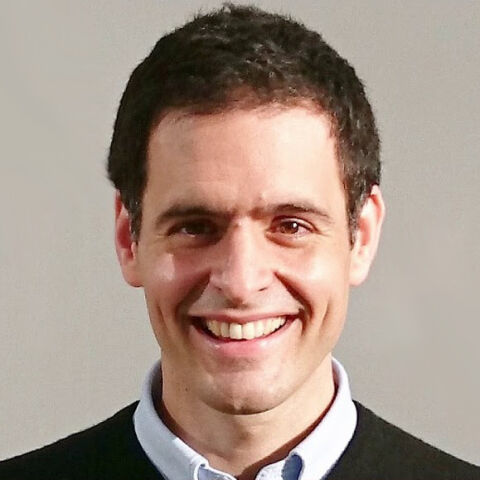
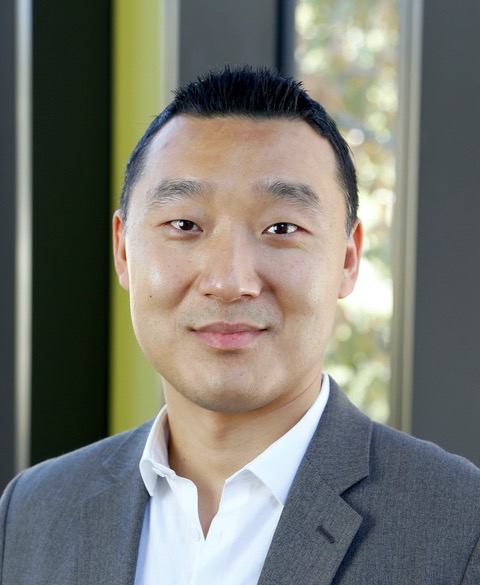
Bio
Yisong Yue is a Professor of Computing and Mathematical Sciences at Caltech and (via sabbatical) a Principal Scientist at Latitude AI. His research interests span both fundamental and applied pursuits, from novel learning-theoretic frameworks all the way to deep learning deployed in autonomous driving on public roads. His work has been recognized with multiple paper awards and nominations, including in robotics, computer vision, sports analytics, machine learning for health, and information retrieval. At Latitude AI, he is working on machine learning approaches to motion planning for autonomous driving.
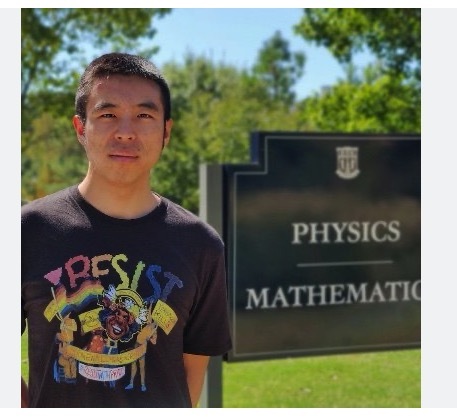
Bio
Didong Li is an Assistant Professor in the Department of Biostatistics in the Gillings School of Global Public Health at the University of North Carolina at Chapel Hill. Before joining UNC Chapel Hill, he was a Postdoctoral Research Associate in the Department of Computer Science at Princeton University working with Barbara Engelhardt and an Assistant Project Scientist in the Department of Biostatistics at University of California, Los Angeles working with Sudipto Banerjee. He was also a Visiting Scientist at the Gladstone Institutes. He completed his Ph.D. in Mathematics at Duke University, supervised by David B. Dunson and Sayan Mukherjee. His research focus is statistical methods development for robust inference of complex and high-dimensional data, specifically covering manifold learning, nonparametric Bayesian inference, information geometry, and spatial statistics. He has applied these methods to electronic healthcare record data, large-scale genetic and survey data, and single-cell RNA-sequencing data. He received the inaugural IMS Lawrence D. Brown Award in 2019.
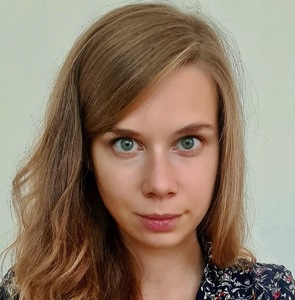
Bio
Natasa is a Senior Machine Learning Scientist at Prescient Design, Genentech since January 2022 where she joined the effort of applying ML to accelerate drug design. Her research interests are related to causal learning, generative models and multiproperty optimization. Before she was a Senior Data Scientist at the Swiss Data Science Center at EPFL-ETHZ where she worked on translational projects applying ML to domain-specific and social science research efforts. She holds a PhD in Data Analytic from University of Lausanne and a BS and MSC in Computer Science and Engineering. During her studies she interend at Facebook (Meta) AI Research and NATO.
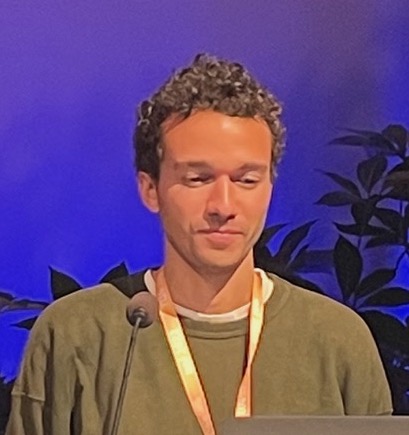
Bio
Yannis Flet-Berliac is a Research Scientist at Instadeep. His interests lie at the intersection of machine learning, healthcare, and safety. He holds a PhD from Inria and completed his postdoc at Stanford University focusing on representation learning, offline reinforcement learning, and deep RL. His work has included applications in Robotics, Healthcare, and Education. Additionally, Yannis holds two MSc degrees from the Technical University of Denmark and École Centrale in France, and has experience as a ML Engineer in various companies and startups, working on projects spanning NLP, Computer Vision, and Speech Processing.
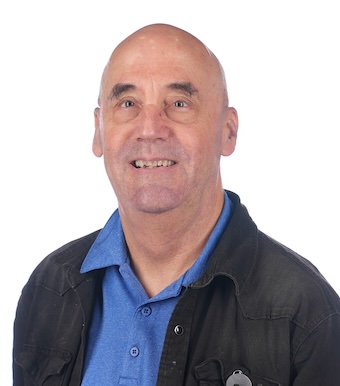
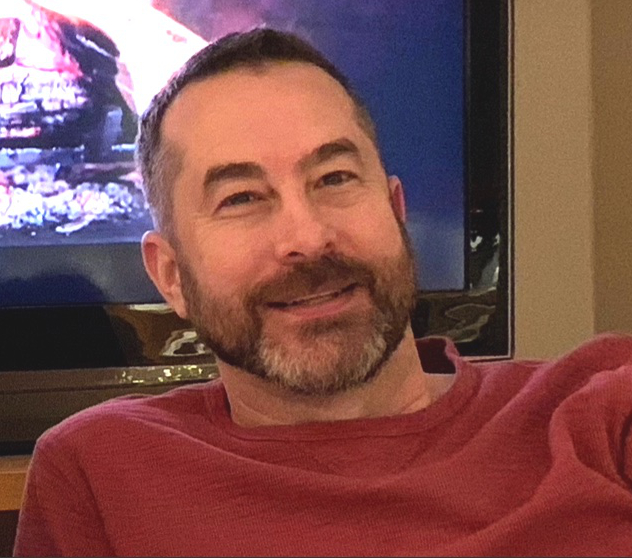
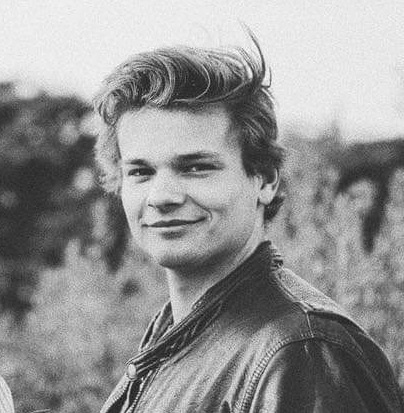
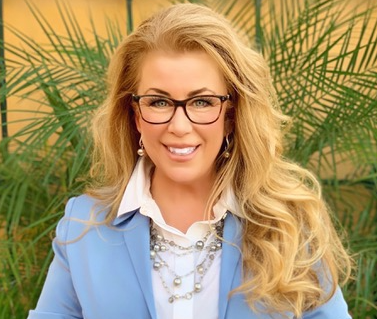