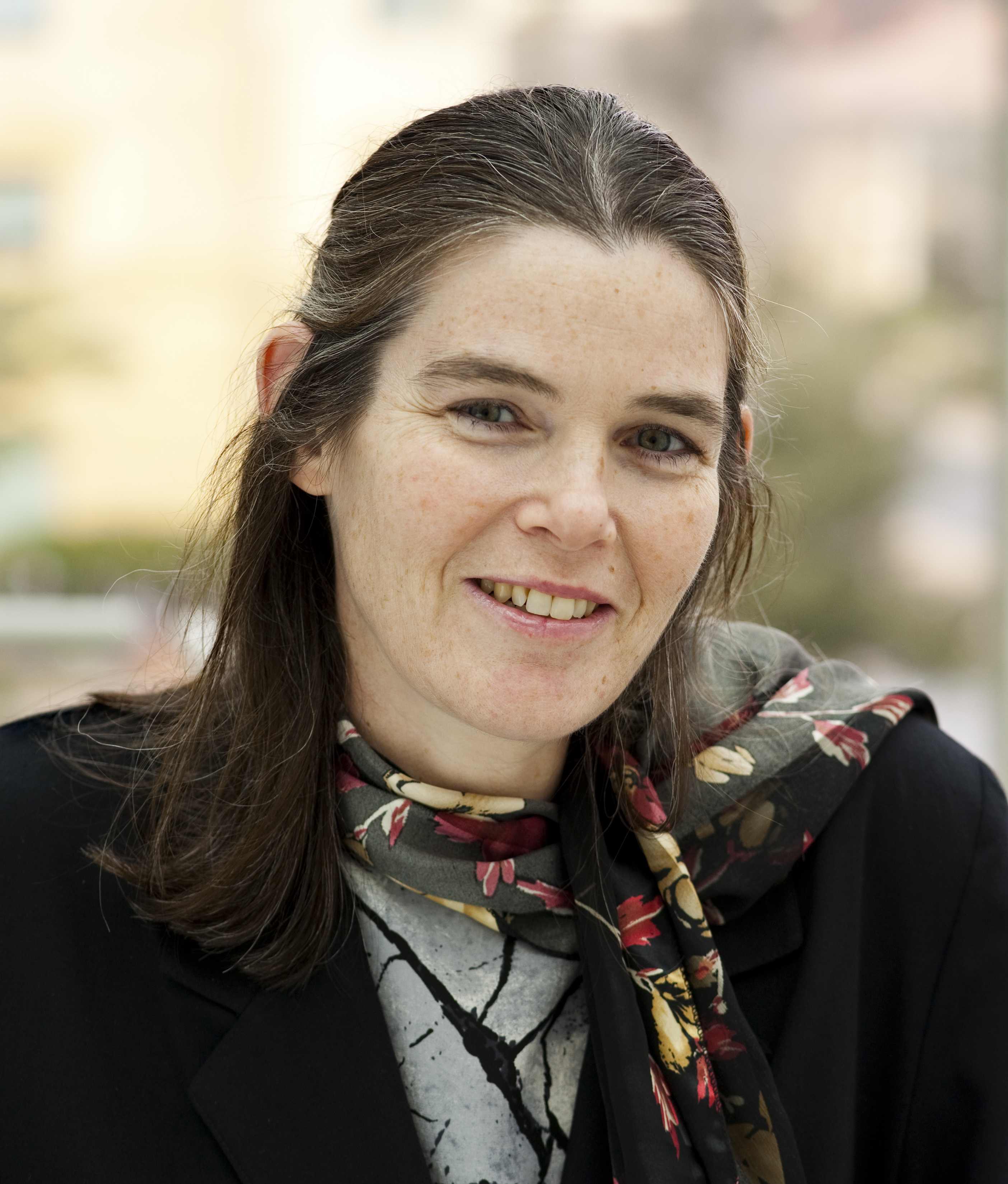
Modern medicine has given us effective tools to treat some of the most significant and burdensome diseases. At the same time, it is becoming consistently more challenging and more expensive to develop new therapeutics. A key factor in this trend is that the drug development process involves multiple steps, each of which involves a complex and protracted experiment that often fails. We believe that, for many of these phases, it is possible to develop machine learning models to help predict the outcome of these experiments, and that those models, while inevitably imperfect, can outperform predictions based on traditional heuristics. To achieve this goal, we are bringing together high-quality data from human cohorts, while also developing cutting edge methods in high throughput biology and chemistry that can produce massive amounts of in vitro data relevant to human disease and therapeutic interventions. Those are then used to train machine learning models that make predictions about novel targets, coherent patient segments, and the clinical effect of molecules. Our ultimate goal is to develop a new approach to drug development that uses high-quality data and ML models to design novel, safe, and effective therapies that help more people, faster, and at a lower cost.
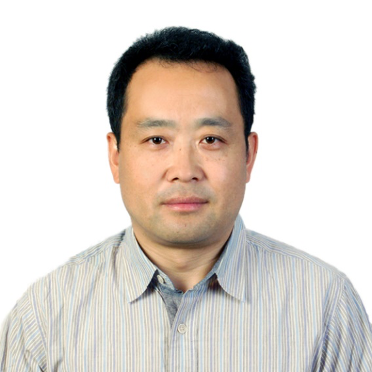
Cryosphere is the layer in a negative temperature state on earth, with continuous distribution and certain thickness. The earth's cryosphere can be divided into three types as continental, marine, and aerial cryosphere, which includes glacier/ice sheet, permafrost, snow cover, lake and river ice, sea ice, ice shelf, iceberg, and solid precipitation, etc. The cryosphere is one of the five major spheres of the climate system. It plays an important role in the earth system with its huge fresh water reserves, latent heat of phase transitions, carbon storage, and unique species habitats and cultural forms.
The presentation starts with an introduction of IPCC main conclusions on human induced climate change and its extremes since the Industrial Involution, especially recent decades. Cryosphere is a sensitive indicator of climate change. The impacts of rapid cryospheric changes have received increasing concerns since 21st century under the background of global warming, extending the research to the interactions between earth’s multi-spheres, including anthroposphere. As a result, cryospheric science has been rapidly developed into a new interdisciplinary, covering its formation, change processes and mechanism, its interactions with and among atmosphere/hydrosphere/biosphere/lithosphere, the influences and adaptations of cryosphere change impacts, the changing functions for serving regional and global economy …
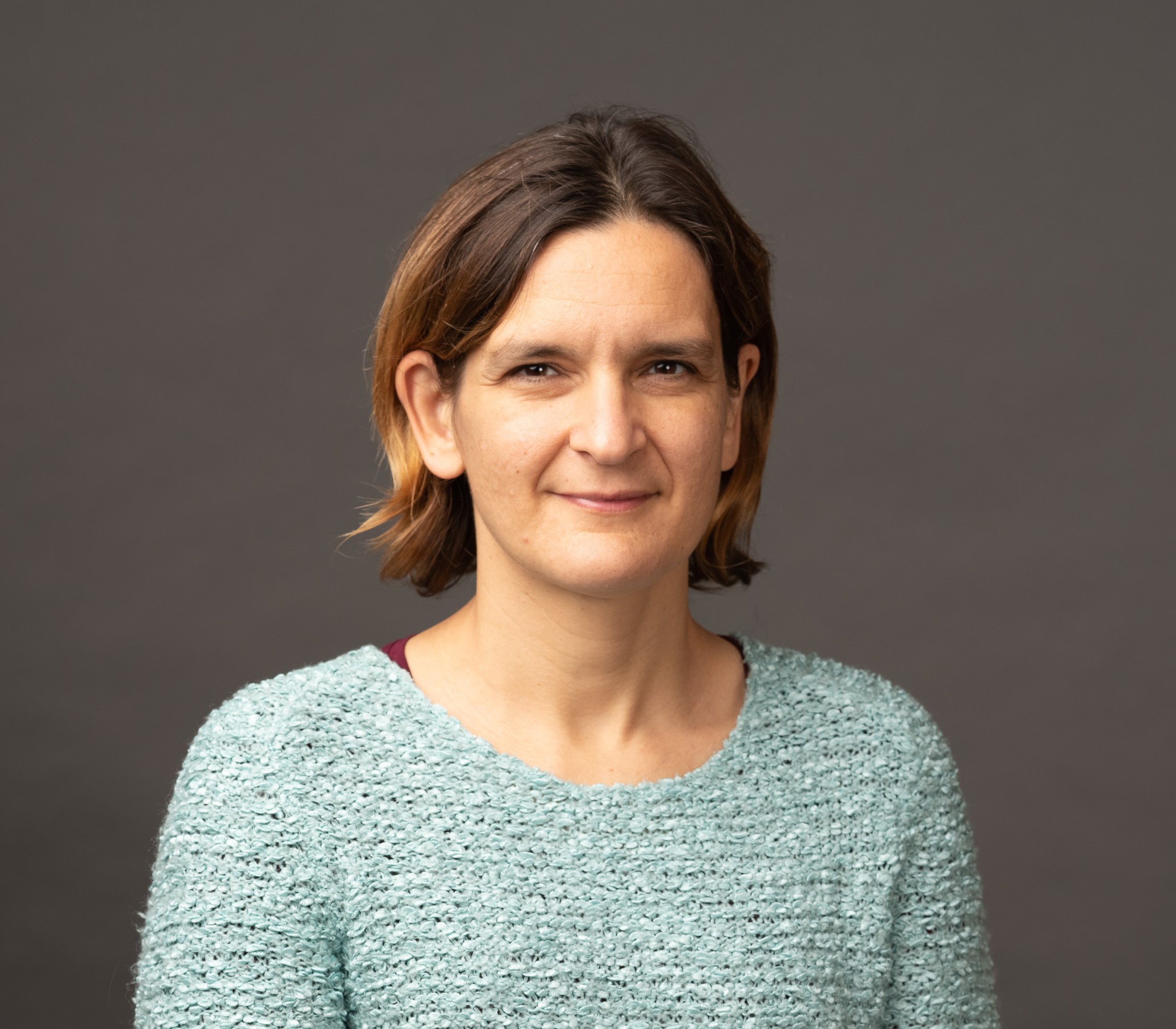
In this talk, I discuss how approaches that may seem very different (randomized controlled trials and Machine Learning) can in fact be complementary. RCT can serve as a useful benchmark to evaluate the real world performance of ML strategies to recover causal effects. ML methods can be used to investigate treatment effect heterogeneity, sort through a large number of possible treatments, etc. The talk concludes with a wish list for Machine learning specialists.

This talk will review recent advances to understand how speech, a unique and defining human behavior, is processed by the cerebral cortex. We will discuss new neuroscientific knowledge on how the brain represents vocal tract movements to give rise to all consonants and vowels, and how this knowledge has been applied to development of a “speech neuroprosthesis” to restore communication for persons living with paralysis.
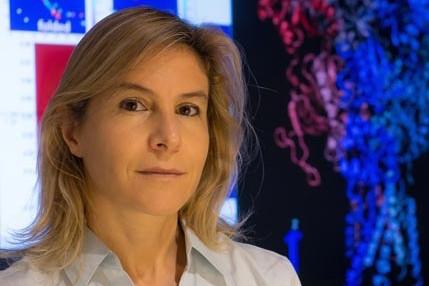
I present an overview of the different ways machine learning is making an impact in molecular science. In particular I focus on theoretical and computational biophysics at the molecular scale, and how machine learning is revolutionizing molecular simulation techniques. I present some of the methods developed in the last few years, the results that have been obtained and the challenges ahead. I describe in some detail the application of machine learning to the development of molecular models for biological macromolecules at resolutions coarser than atomistic, that can accurately reproduce the behavior of the system as described by atomistic models or experimental measurements.