Invited Talks
Modern medicine has given us effective tools to treat some of the most significant and burdensome diseases. At the same time, it is becoming consistently more challenging and more expensive to develop new therapeutics. A key factor in this trend is that the drug development process involves multiple steps, each of which involves a complex and protracted experiment that often fails. We believe that, for many of these phases, it is possible to develop machine learning models to help predict the outcome of these experiments, and that those models, while inevitably imperfect, can outperform predictions based on traditional heuristics. To achieve this goal, we are bringing together high-quality data from human cohorts, while also developing cutting edge methods in high throughput biology and chemistry that can produce massive amounts of in vitro data relevant to human disease and therapeutic interventions. Those are then used to train machine learning models that make predictions about novel targets, coherent patient segments, and the clinical effect of molecules. Our ultimate goal is to develop a new approach to drug development that uses high-quality data and ML models to design novel, safe, and effective therapies that help more people, faster, and at a lower cost.

Daphne Koller
Daphne Koller is CEO and Founder of insitro, a machine-learning enabled drug discovery company transforming the way drugs are discovered and delivered to patients. She is the co-founder of online education platform Engageli and of Coursera, the largest platform for massive open online courses (MOOCs), where she was co-CEO and President. Daphne was the Rajeev Motwani Professor of Computer Science at Stanford University, where she served on the faculty for 18 years. She has also been Chief Computing Officer of Calico, an Alphabet company in the healthcare space. She is the author of over 200-refereed publications appearing in venues such as Science, Cell, and Nature Genetics. Daphne was recognized as one of TIME Magazine¹s 100 most influential people in 2012 and Newsweek¹s 10 most important people in 2010. She has been honored with multiple awards and fellowships during her career including the Sloan Foundation Faculty Fellowship in 1996, the ONR Young Investigator Award in 1998, the Presidential Early Career Award for Scientists and Engineers (PECASE) in 1999, the IJCAI Computers and Thought Award in 2001, the MacArthur Foundation Fellowship in 2004, and the ACM Prize in Computing in 2008. Daphne was inducted into the National Academy of Engineering in 2011 and elected a fellow of the American Association for Artificial Intelligence in 2004, the American Academy of Arts and Sciences in 2014 and of the International Society of Computational Biology in 2017. Her teaching was recognized via the Stanford Medal for Excellence in Fostering Undergraduate Research, and as a Bass University Fellow in Undergraduate Education.
Cryosphere is the layer in a negative temperature state on earth, with continuous distribution and certain thickness. The earth's cryosphere can be divided into three types as continental, marine, and aerial cryosphere, which includes glacier/ice sheet, permafrost, snow cover, lake and river ice, sea ice, ice shelf, iceberg, and solid precipitation, etc. The cryosphere is one of the five major spheres of the climate system. It plays an important role in the earth system with its huge fresh water reserves, latent heat of phase transitions, carbon storage, and unique species habitats and cultural forms.
The presentation starts with an introduction of IPCC main conclusions on human induced climate change and its extremes since the Industrial Involution, especially recent decades. Cryosphere is a sensitive indicator of climate change. The impacts of rapid cryospheric changes have received increasing concerns since 21st century under the background of global warming, extending the research to the interactions between earth’s multi-spheres, including anthroposphere. As a result, cryospheric science has been rapidly developed into a new interdisciplinary, covering its formation, change processes and mechanism, its interactions with and among atmosphere/hydrosphere/biosphere/lithosphere, the influences and adaptations of cryosphere change impacts, the changing functions for serving regional and global economy and society. Cryospheric science is an inevitable scope of international research on the earth and environmental changes, as well as on human sustainable development.
The study on Chinese cryosphere has been developing rapidly following the scope of Cryospheric Science in the past 20 years, especially in the last decade. It has presented systematic achievements in terms of changes in the cryosphere and their impacts on ecology, hydrology, climate, environment, society and economy, and also obtain systematic understanding of the connotation and extension of the Cryospheric Science, made important contributions to the establishment and development of research framework and disciplinary system of the cryospheric science.
The presentation will also show some case studies on cryosphere using machine learning, such as data mining, permafrost mapping and soil organic carbon estimation, Arctic sea ice prediction, outlet glacier instability estimation of ice sheet, as well as paleoclimatic proxy reconstructions. Machine learning is a promising tool for studying both natural aspects and the socioeconomic aspects when studying cryospheric impacts such as services and hazards. There are complex linkages between cryospheric impacts and UN 2030s’ Sustainable Development Goals (SDGs) over the cryospheric influential regions, it is promising to use big data and machine learning to deepen our knowledge.
Key words: IPCC, cryospheric science, sustainable development, machine learning
Qin Dahe
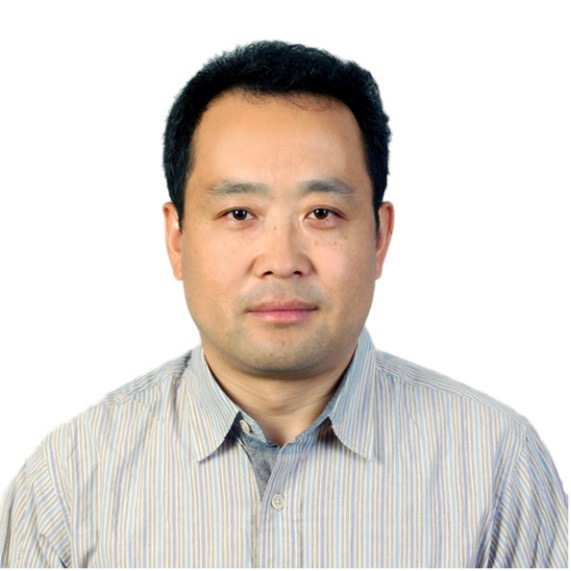
Xiao Cunde
Dr. Cunde Xiao is the Director of State Key Laboratory of Earth Surface Processes and Resources Economy, Beijing Normal University, China. He graduated from Lanzhou University (China) in 1992, and received Ph.D on glaciology in 1997. He has worked in the fields of polar glaciology and meteorology since then. His major research focus has been ice core studies relating to paleoclimate and paleoenvironment, and present-day cold region meteorological and glaciological processes that impact environmental and climatic changes, recently more on cryospheric functions and their socioeconomic services. Dr. Xiao is the former Vice-president of International Association of Cryospheric Sciences (IACS), IUGG; Review editor of both IPCC AR5 WG1 and Special Report of Ocean and Cryosphere under Changing Climate (SROCC), Council member of International Glaciology Society (IGS), member of the Steering Committee for the international program, Antarctica in the Global Climate System (AGCS) of the Scientific Committee on Antarctica Research (SCAR), member of the Scientific Steering Committee, of the World Climate Research Programme (WCRP)– Climate and the Cryosphere initiative (CliC), member of Expert Committee of Polar and High Mountain Observation, Research and Services (EC-PHORS), WMO. He is now Coordinating Lead Author (CLA) of Chapter 9, IPCC AR6 WG1; He has published more than 170 scientific papers.
In this talk, I discuss how approaches that may seem very different (randomized controlled trials and Machine Learning) can in fact be complementary. RCT can serve as a useful benchmark to evaluate the real world performance of ML strategies to recover causal effects. ML methods can be used to investigate treatment effect heterogeneity, sort through a large number of possible treatments, etc. The talk concludes with a wish list for Machine learning specialists.
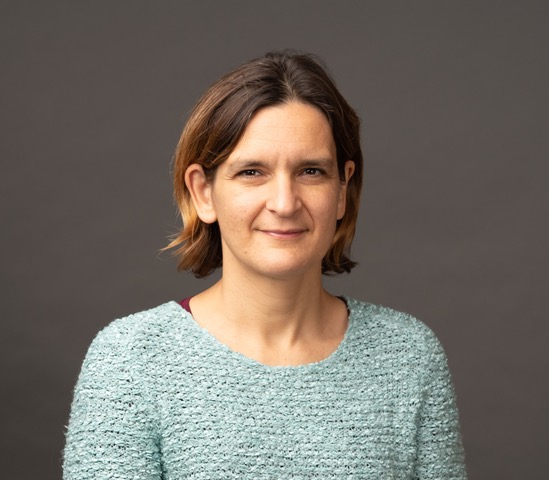
Esther Duflo
Esther Duflo is the Abdul Latif Jameel Professor of Poverty Alleviation and Development Economics in the Department of Economics at the Massachusetts Institute of Technology and a co-founder and co-director of the Abdul Latif Jameel Poverty Action Lab (J-PAL). In her research, she seeks to understand the economic lives of the poor, with the aim to help design and evaluate social policies. She has worked on health, education, financial inclusion, environment and governance.
Professor Esther Duflo’s first degrees were in history and economics from Ecole Normale Superieure, Paris. She subsequently received a Ph.D. in Economics from MIT in 1999.
Duflo has received numerous academic honors and prizes including 2019 Sveriges Riksbank Prize in Economic Sciences in Memory of Alfred Nobel (with co-Laureates Abhijit Banerjee and Michael Kremer), the Princess of Asturias Award for Social Sciences (2015), the A.SK Social Science Award (2015), Infosys Prize (2014), the David N. Kershaw Award (2011), a John Bates Clark Medal (2010), and a MacArthur “Genius Grant” Fellowship (2009). With Abhijit Banerjee, she wrote Poor Economics: A Radical Rethinking of the Way to Fight Global Poverty, which won the Financial Times and Goldman Sachs Business Book of the Year Award in 2011 and has been translated into more than 17 languages, and the recently released Good Economics for Hard Times.
Duflo is the Editor of the American Economic Review, a member of the National Academy of Sciences and a Corresponding Fellow of the British Academy.
This talk will review recent advances to understand how speech, a unique and defining human behavior, is processed by the cerebral cortex. We will discuss new neuroscientific knowledge on how the brain represents vocal tract movements to give rise to all consonants and vowels, and how this knowledge has been applied to development of a “speech neuroprosthesis” to restore communication for persons living with paralysis.
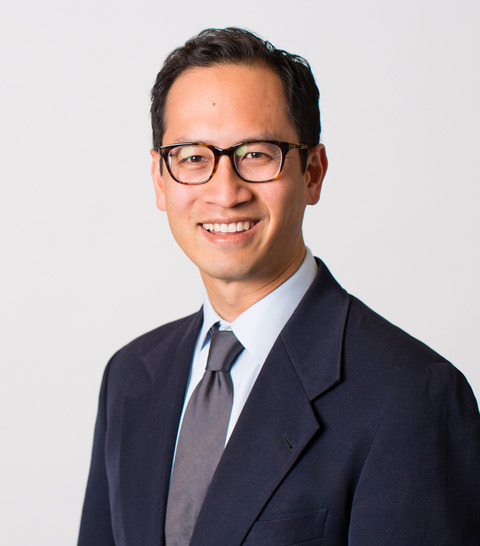
Edward Chang
I present an overview of the different ways machine learning is making an impact in molecular science. In particular I focus on theoretical and computational biophysics at the molecular scale, and how machine learning is revolutionizing molecular simulation techniques. I present some of the methods developed in the last few years, the results that have been obtained and the challenges ahead. I describe in some detail the application of machine learning to the development of molecular models for biological macromolecules at resolutions coarser than atomistic, that can accurately reproduce the behavior of the system as described by atomistic models or experimental measurements.
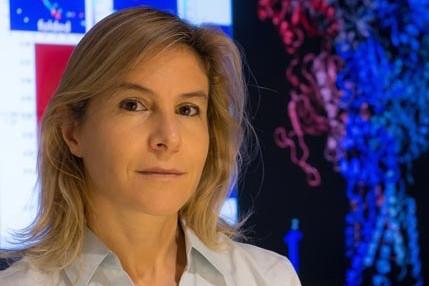
Cecilia Clementi
Cecilia Clementi is a Professor of Chemistry, and Chemical and Biomolecular Engineering, and Senior Scientist in the Center for Theoretical Biological Physics at Rice University. Cecilia received her Laurea (B.S.) degree in Physics from the University of Florence, Italy, in 1995, and her PhD in Physics from the International School for Advanced Studies (SISSA/ISAS) in Trieste, Italy, in 1998. After a postdoctoral fellowship in the La Jolla Interfaces in Science (LJIS) program at the University of California San Diego, she joined the Rice faculty in 2001, where she leads an interdisciplinary group working on multiscale macromolecular modeling. Cecilia's work has been recognized with multiple awards, such as the Norman Hackerman Award in Chemical Research of the Welch Foundation, and the NSF-CAREER award.
Cecilia is serving as co-Director of the NSF-funded Molecular Sciences Software Institute (MolSSI), where she is responsible for the activities in biomolecular simulation and in international engagement. She is also an Einstein Visiting Fellow at the Freie Universitat in Berlin, Germany.