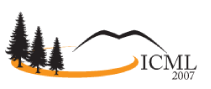
|
|
|
Alan
Fern
afern@eecs.orst.edu
1148,
Kelly Engineering Center
Corvallis, OR 97333
(541) 737-9202
|
|
|
ICML 2007
WORKSHOPS: June 24, 2007
|
Chair: Alan Fern
|
There will be 4 workshops held at the end of
ICML-2007 on Sunday, June 24, 2007 at Oregon
State University, Corvallis, Oregon,
U.S.A.
Please refer to the workshop web-pages below
regarding submission deadlines and formats.
Workshop participants will not be given workshop proceedings in
a hardcopy format. Instead, organizers of the workshops will make the
proceedings available on their website prior to the conference.
Accepted Workshops
- Challenges
and
Applications of Grammar Induction (CAGI'07)-- Grammar
Induction (GI), also known as Grammatical
Inference, is about learning grammars from data. A well-known important
application of GI is natural language learning, but it is applicable in
a much broader sense to the problem of learning structural models from
data. The CAGI workshop aims at highlighting current challenges in GI
with a special focus on applicability issues including. See web-site
for more details.
- Induction
of Process Models-- This workshop focuses on
learning process models from observations and background knowledge.
Although a large literature on time-series
analysis exists, it emphasizes descriptive models that ignore the
underlying mechanisms of the studied system. In contrast, the workshop
centers on knowledge-aware learning approaches that refer to relevant
scientific concepts. These methods work to uncover the mechanisms that
generate observed behavior, to identify plausible state-transition
networks, or to induce pathways that depict flux within the
system. The resulting models may characterize behavior in
qualitative, quantitative, or mixed representations with an emphasis on
comprehensibility. Key research directions in process-based
modeling include knowledge-rich induction, learning in expressive
languages, and modeling temporal and spatial data. See web-site for
more details.
- Constrained
Optimization and Learning with
Structured Outputs-- In recent years, there
has been a great deal of work relating
constrained optimization problems with machine learning in structured
output spaces. This has given rise to a number of novel and powerful
approaches to solving some extremely difficult machine learning
problems. The objective of this workshop is to bring together
researchers in both of these areas, thereby encouraging
furthercollaboration and increasing awareness of the issues at hand in
both communities. See web-site for more details.
|